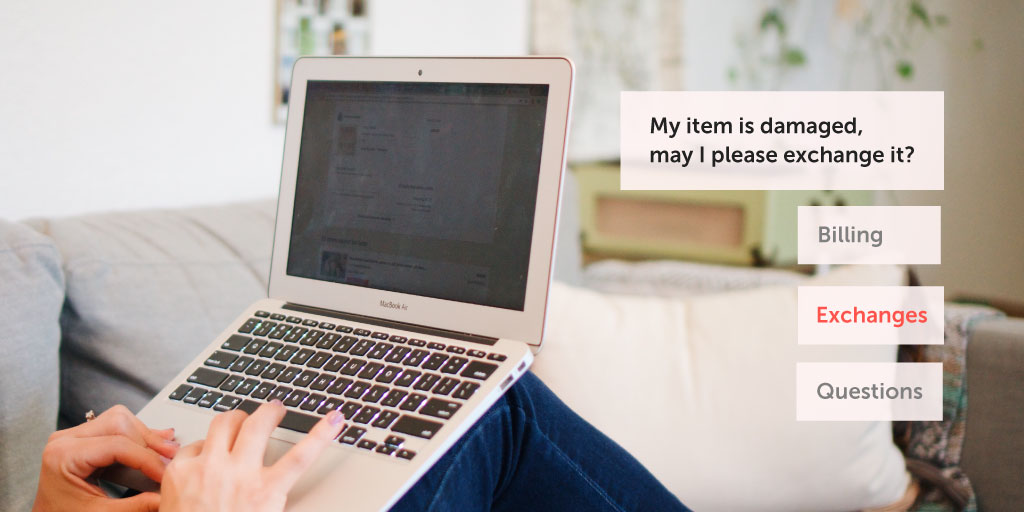
If you have ever been drawn to the words “artificial intelligence” in a sales pitch or wondered if machine learning could improve your business, you might have also questioned if your use case is even a good fit for what a machine learning model can do.
A good way to approach answering this question is to consider the data you need to provide this artificially intelligent system in order for it to produce the promised result in customer experience. That sounds like a reasonable exchange, except, are you sure you know what data means in this context?
What Is Data?
Let’s say you are interested in a machine learning model that can predict the contact reason for inbound support emails (surprise: this is Kustomer IQ!). The model needs to see examples of previous support conversations and how they were labeled in order to learn what to do when new, unlabeled emails show up in the future. These examples are your data: a collection of paired inputs and outputs from which a machine learning model will extract patterns to make predictions.

In this case, it’s up to you to determine the inputs (“Inbound Support Email”) and outputs (“Contact Reason”) and which input-output pairings best represent the predictions you want the model to make. And in general terms, the more data you expose the machine to, the smarter it gets.
Make sense? This is data. Without data, machine learning models can’t work. And a machine learning model will only learn from what’s in the training data: what doesn’t appear explicitly in the dataset will remain unknown to the system.
Data Quality in Customer Service
As we said before, the models extract patterns in order to apply outputs. You’ll notice in the above diagram, we did not tell the model how we were able to determine Contact Reason. We are just planning to show input-output examples and trust it will eventually come up with a way to infer “Contact Reason” from an “Inbound Support Email”. This is where data quality becomes important: if we give the model poor-quality data, the model will make bad patterns and ultimately incorrect predictions.
Poor quality data is noisy, conflicting, or perhaps consists of too few examples. In the diagram above, the last Inbound Support Email has been categorized as an “Exchange”. We already mentioned that it’s up to you to determine the output in the training dataset, so you or one of your agents decided the contact reason was an exchange. What if another agent decided the contact reason was “Return”? Technically, a return might need to happen for an exchange to occur, so the agent felt the return categorization made sense.
Now, what if your agents made this mistake 50% or 25% of the time? This means your resulting training data does not reflect the truth of what you want the model to predict — sometimes the conversation is labeled as an “Exchange” and sometimes the same type of conversation is labeled as “Return”, but it should always be Exchange. In this case, the model will extract a pattern that might not consistently lead it to infer your preferred contact reason for future inbound support emails.
This is an example of one of the main causes of poor-quality data: unclear and conflicting boundaries between categories or topics.
Here are the characteristics your data needs to have to be considered “high quality” by Kustomer IQ standards:
- Heterogeneity (this is demonstrated in the scenario above): Clear and distinct topic boundaries between different tags and attributes
- Stability: No big re-categorization changes 60-90 days prior to model training
- Volume: Between 5,000 – 7,000 email conversations from the previous month, or about 500 conversations per topic
Recommendations for Creating High-Quality Data for Kustomer IQ
Kustomer IQ relies on machine learning models to predict customer contact reasons and suggests conversation shortcuts to agents. It’s important for you to have high-quality data to make these models work as expected.
There are a number of steps you can take to ensure the highest quality of your data and leverage automation from the very beginning. Here are our recommendations:
- Design tags and custom fields that best suit your needs. While it’s impossible to cover all cases, try to focus on the most important ones in terms of expected volume. For example, order status, returns & refunds, or promo codes.
- Create new tags to cover unlabelled information rather than redefining and remapping everything from scratch.
- Add granularity in an incremental fashion. When possible, start with a broad category, such as pets, before attempting to split it into various, more specific categories, such as cats, dogs, or birds.
- Try to use clearly defined tags and categories to organize your information. A good rule of thumb is to test them with people. If your team understands their boundaries correctly and knows when to apply them, a machine learning algorithm will be able to figure out their meanings.
- Try to make your team work as consistently as possible. Again, this is easier to achieve when tag limits are clear.
- Try to keep data stable for a while so that you can grow the number of examples using your tags and custom fields. While you can iterate and re-define your tags and custom fields at any time, regularly changing this information will affect the amount of data points you get. Also, keep in mind that any re-definition of tags and categories will need the model to be re-trained.
Elevate Your Overall CX With Kustomer
Data analytics is just one of many tools for providing great customer service. If you’re looking for further support on how to create the best customer experience for your clients with a customer service software solution that includes live chat, book a demo today.